why Facebook Message Meta Ai Image Not Working? In a world where technological advancements are shaping our daily lives, the field of artificial intelligence (AI) has been at the forefront of innovation. Meta (formerly Facebook) has been a major player in this arena, investing heavily in AI research and development.
However, despite their efforts, Meta’s AI image recognition technology has been facing significant challenges, leading to widespread concerns about its effectiveness. This article delves into the reasons behind the failure of Meta’s AI image technology and explores the potential implications for the company and the broader AI community.
The Promise of Meta’s AI Image Technology
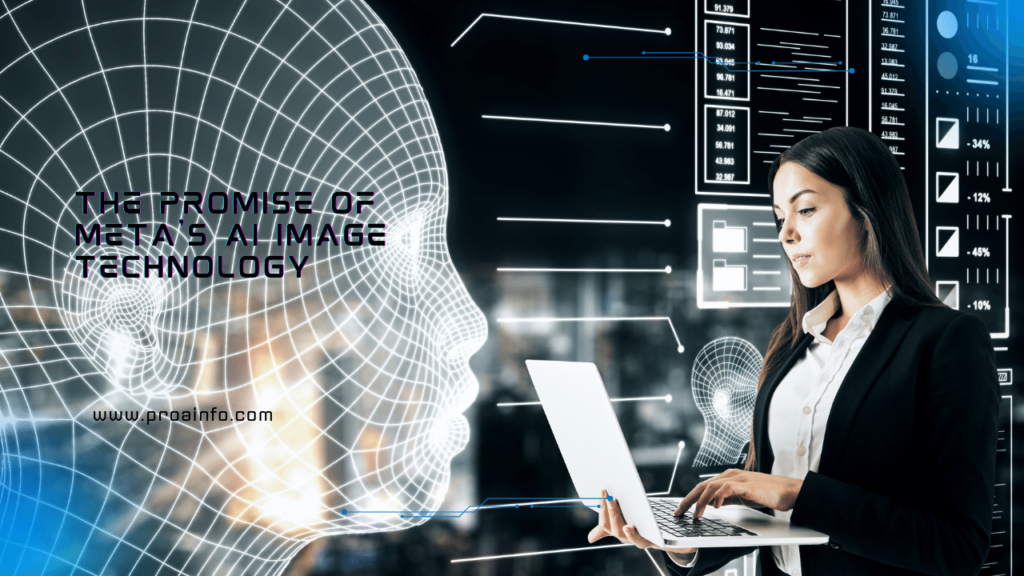
- Meta’s Vision for AI Image Recognition
- The Potential Applications and Benefits
- Expectations and Hype Surrounding the Technology
Meta’s ambitious vision for AI image recognition technology was driven by the vast potential applications and benefits it could bring. From enhancing user experiences on social media platforms to revolutionizing industries like healthcare, security, and retail, the possibilities seemed endless. The company invested heavily in research and development, assembling a team of top AI experts and leveraging its massive data resources.
The promise of Meta’s AI image technology was met with considerable hype and expectations. Users anticipated seamless image recognition capabilities, enabling features like automated content moderation, personalized advertising, and advanced image search functionalities. Businesses and organizations eagerly awaited the technology’s integration into their operations, envisioning improved efficiency, cost savings, and enhanced customer experiences.
The Reality: Meta’s AI Image Technology Falls Short
- Accuracy and Reliability Issues
- Bias and Discrimination Concerns
- Scalability and Performance Limitations
However, despite the high hopes and substantial investments, Meta’s AI image technology has failed to deliver on its promises. Reports have surfaced highlighting significant accuracy and reliability issues, casting doubt on the technology’s practical applications.
One of the major concerns is the system’s inability to consistently and accurately recognize and classify images across diverse scenarios. From misidentifying objects and individuals to failing to detect explicit or harmful content, the AI’s shortcomings have raised serious questions about its efficacy.
Moreover, there have been numerous instances of the AI exhibiting biases and discrimination, particularly in the context of race, gender, and other demographic factors. These concerning tendencies have sparked debates about the ethical implications of deploying such technologies and the potential for perpetuating societal biases.
Furthermore, scalability and performance limitations have emerged as significant roadblocks. As the volume and complexity of image data continue to grow exponentially, Meta’s AI image technology has struggled to keep pace, leading to slower processing times, increased computational costs, and decreased overall efficiency.
The Root Causes of Meta’s AI Image Technology Failure
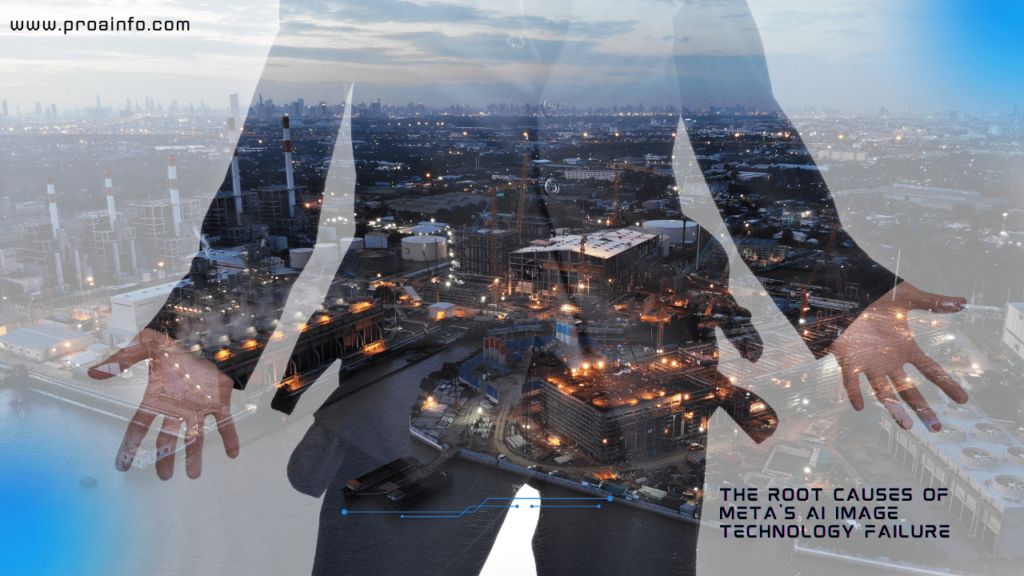
- Data Quality and Diversity Issues
- Algorithmic Limitations and Complexity
- Human Oversight and Accountability Challenges
- Ethical Considerations and Societal Impacts
To understand the root causes of Meta’s AI image technology failure, it is crucial to examine the underlying factors that have contributed to its shortcomings.
- Data Quality and Diversity Issues: One of the fundamental challenges lies in the quality and diversity of the training data used to develop the AI models. Despite Meta’s access to vast amounts of data, the lack of comprehensive and representative datasets spanning diverse demographics, cultures, and contexts has likely hindered the AI’s ability to generalize and perform accurately across different scenarios.
- Algorithmic Limitations and Complexity: The complexity of image recognition tasks, coupled with the limitations of current AI algorithms and architectures, has posed significant challenges. While advancements have been made in areas like deep learning and computer vision, the intricate nature of visual perception and the nuances of human interpretation have proved difficult for AI systems to replicate effectively.
- Human Oversight and Accountability Challenges: The development and deployment of AI systems require careful human oversight and accountability measures. However, the rapid pace of AI advancements and the scale of Meta’s operations may have contributed to lapses in monitoring and quality control, leading to unintended biases and errors in the AI image technology.
- Ethical Considerations and Societal Impacts: The failure of Meta’s AI image technology also raises important ethical questions and highlights the potential societal impacts of deploying such technologies prematurely or without proper safeguards. Concerns about privacy, discrimination, and the amplification of existing biases have sparked debates about the responsible development and use of AI systems.
Implications and Consequences
- Reputational Damage and Loss of Trust
- Competitive Disadvantage
- Regulatory Scrutiny and Compliance Challenges
- Ethical and Social Responsibilities
The failure of Meta’s AI image technology has far-reaching implications and consequences for the company and the broader AI community.
- Reputational Damage and Loss of Trust: Meta’s inability to deliver on its promises regarding AI image recognition has dealt a significant blow to its reputation as a technology leader. The public’s trust in the company’s AI capabilities has been eroded, potentially impacting user engagement and stakeholder confidence.
- Competitive Disadvantage: In the highly competitive technology landscape, Meta’s setback with AI image technology could provide an opening for rivals to gain a competitive edge. Companies that successfully develop and implement advanced image recognition capabilities may attract more users, partners, and investment opportunities.
- Regulatory Scrutiny and Compliance Challenges: As AI technologies become more pervasive, regulatory bodies are increasingly focused on ensuring their safe and ethical deployment. Meta’s struggles with AI image technology could attract heightened scrutiny from regulators, potentially leading to stricter compliance requirements and oversight measures.
- Ethical and Social Responsibilities: The failure of Meta’s AI image technology also highlights the company’s ethical and social responsibilities. As a technology leader with significant influence, Meta must prioritize addressing the biases, discrimination, and potential negative impacts of its AI systems on society.
Moving Forward: Lessons and Recommendations
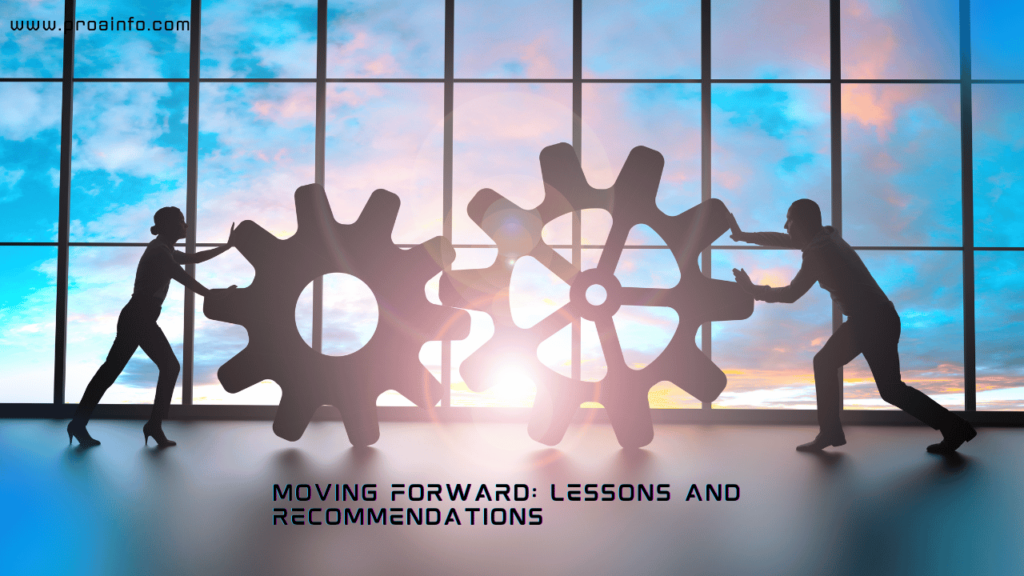
- Strengthening Data Quality and Diversity
- Investing in Advanced Algorithms and Architectures
- Embracing Transparency and Accountability
- Prioritizing Ethical AI Development
- Collaborative Efforts and Knowledge Sharing
To overcome the challenges and regain momentum in the AI image recognition domain, Meta and the broader AI community must learn from this experience and implement strategic measures.
- Strengthening Data Quality and Diversity: Addressing the root cause of data quality and diversity issues is crucial. Meta should prioritize curating comprehensive and representative datasets that capture the nuances of diverse demographics, cultures, and contexts. Collaborating with experts, communities, and stakeholders can help ensure a more inclusive and accurate data foundation.
- Investing in Advanced Algorithms and Architectures: Continuous research and development efforts are essential to push the boundaries of AI algorithms and architectures. By investing in cutting-edge techniques, such as federated learning, transfer learning, and multi-modal approaches, Meta may be able to overcome the current limitations and improve the accuracy and reliability of its AI image technology.
- Embracing Transparency and Accountability: Fostering transparency and accountability throughout the AI development lifecycle is crucial for building trust and addressing potential biases and errors. Meta should establish robust monitoring and quality control measures, engage in regular audits, and involve diverse stakeholders in the decision-making processes.
- Prioritizing Ethical AI Development: Meta must prioritize the ethical development and deployment of AI systems, ensuring that they align with societal values and mitigate potential negative impacts. This involves implementing rigorous ethical frameworks, conducting thorough risk assessments, and collaborating with experts in fields such as ethics, law, and social sciences.
- Collaborative Efforts and Knowledge Sharing: The challenges faced by Meta’s AI image technology underscore the importance of collaborative efforts and knowledge sharing within the AI community. By fostering open dialogue, sharing insights and best practices, and engaging in cross-disciplinary collaborations, the AI community can collectively address the complexities of image recognition and advance the field in a responsible and ethical manner.
Conclusion
The failure of Meta’s AI image technology serves as a stark reminder of the complexities and challenges inherent in the development and deployment of advanced AI systems. While the promise of AI-driven image recognition holds immense potential, the journey towards realizing that potential is fraught with obstacles.
As Meta navigates this setback, it must learn from the root causes and embrace a multi-faceted approach to address the issues of data quality, algorithmic limitations, human oversight, and ethical considerations. By prioritizing transparency, accountability, and responsible AI development, the company can regain public trust and work towards creating AI image technology that is accurate, reliable, and aligned with societal values.
Moreover, this experience highlights the need for collaborative efforts and knowledge sharing within the AI community. By fostering open dialogue, cross-disciplinary collaborations, and a commitment to ethical AI development, the industry can collectively overcome the challenges and unlock the true potential of AI-driven image recognition.
Ultimately, the failure of Meta’s AI image technology serves as a catalyst for introspection, innovation, and a renewed commitment to responsible AI development. As the world continues to embrace the transformative power of artificial intelligence, it is crucial that companies like Meta lead by example, prioritizing accuracy, fairness, and ethical considerations in their pursuit of technological advancements.
FAQs
What is Meta’s AI image technology?
Meta’s AI image technology is an artificial intelligence system developed by the company (formerly known as Facebook) to recognize, classify, and interpret images and visual content. It was designed to enhance user experiences and enable advanced image-related features across Meta’s platforms and services.
What are the root causes of these failures?
The root causes include data quality and diversity issues, algorithmic limitations and complexity, challenges with human oversight and accountability, and ethical considerations related to the societal impacts of deploying such technologies prematurely or without proper safeguards.
What are the implications of Meta’s AI image technology failure?
The failure has led to reputational damage and loss of trust in Meta’s AI capabilities, potential competitive disadvantages, increased regulatory scrutiny and compliance challenges, and heightened concerns about the ethical and social responsibilities of deploying AI systems.
How can Meta address these issues?
Meta can address these issues by strengthening data quality and diversity, investing in advanced algorithms and architectures, embracing transparency and accountability, prioritizing ethical AI development, and fostering collaborative efforts and knowledge sharing within the AI community.
What are the broader implications for the AI industry?
The failure of Meta’s AI image technology highlights the importance of responsible AI development, addressing biases and ethical concerns, fostering transparency and accountability, and prioritizing accuracy and reliability over hype and unrealistic expectations.
How can users or organizations mitigate the risks associated with AI image technology?
Users and organizations can mitigate risks by being cautious about relying solely on AI image technology for critical applications, implementing human oversight and validation processes, and staying informed about the limitations and potential biases of such systems.
What role can regulations and governance play in addressing AI image technology challenges?
Regulations and governance can play a crucial role in establishing guidelines, standards, and accountability measures for the development and deployment of AI image technology. This can help ensure ethical and responsible practices, protect user privacy and rights, and mitigate potential societal harms.
How can the AI community collaborate to overcome these challenges?
The AI community can collaborate by fostering open dialogue, sharing insights and best practices, engaging in cross-disciplinary research and collaborations, and prioritizing ethical AI development aligned with societal values and principles.
5 thoughts on “why Facebook Message Meta Ai Image Not Working?”